Million Concepts is a
full-stack software development, research, and consulting
company with special expertise in remote sensing, big data,
and interaction design for domain experts. We mostly solve
hard problems in space, but we like to solve them on Earth too.
We offer custom solutions that combine approaches from science,
business, design, and ethnography. We will immerse ourselves in
your world, learn about your problems, and fix them.
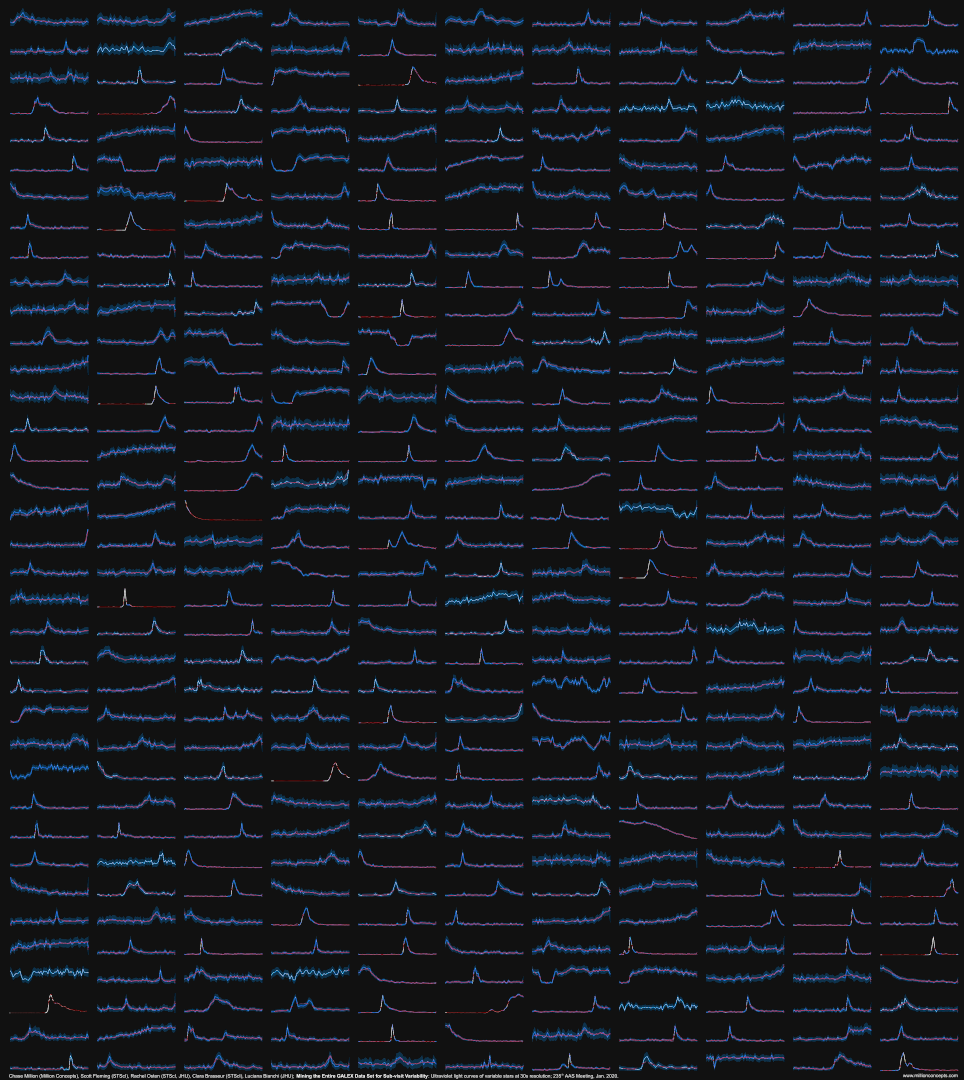